INM-4’s NIDS Group Presents Breakthrough in EEG Analysis at ICLR 2025
20th April 2025
We are pleased to announce that our latest work has been selected for presentation at the International Conference on Learning Representations (ICLR) 2025, one of the premier conferences in the field of artificial intelligence. The conference will take place from April 24 to April 28, 2025, in Singapore.
In this study, researchers from the Neuro Imaging Data Science (NIDS) group at INM-4, Forschungszentrum Jülich, introduce three novel algorithms that extend the transformer architecture for electroencephalography (EEG) signal processing. Our approach addresses key challenges in neural signal interpretation by tightly integrating explainable artificial intelligence (XAI) into the model design.
Unlike conventional uses of XAI as a post-hoc interpretability layer, this method embeds XAI mechanisms directly into the training process to actively guide learning. This results in significantly reduced overfitting to spurious or irrelevant EEG features—an issue that has long hindered the application of standard transformer models to neural data.
Presenter, Hanning Guo explained, "This work highlights the potential of combining deep learning with interpretable model design to advance robust, trustworthy brain signal analysis and contributes to the broader goal of explainable neuro-AI."
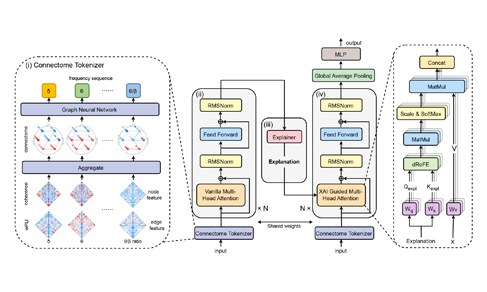
The diagram above shows the architecture of XAIguiFormer. The XAIguiFormer forward process can be described as follows: (i) construct multi-frequency band connectomes and generate a sequence in the frequency domain by connectome tokeniser, (ii) forward pass the frequency sequence by vanilla transformer,(iii) obtain refined features by explaining the vanilla transformer, (iv) feedforward refined features and the frequency sequence through the XAI guided transformer. Subsequently, the MLP is employed as the classification head to predict the label of the brain disorder.
Origional confrence abstract - XAIguiFormer: explainable artificial intelligence guided transformer for brain disorder identification
Hanning Guo, Farah Abdellatif, Yu Fu, N. Jon Shah, Abigail Morrison, Jürgen Dammers