Deep learning-based classification
We propose an artifact classification scheme based on a combined deep and convolutional neural network (DCNN) model, to automatically identify cardiac and ocular artifacts from neuromagnetic data, without the need for additional electrocardiogram (ECG) and electrooculogram (EOG) recordings. From independent components, the model uses both the spatial and temporal information of the decomposed magnetoencephalography (MEG) data. In total, 7122 samples were used after data augmentation, in which task and nontask related MEG recordings from 48 subjects served as the database for this study. Artifact rejection was applied using the combinedmodel,which achieved a sensitivity and specificity of 91.8% and 97.4%, respectively.The overall accuracy of the model was validated using a cross-validation test and revealed a median accuracy of 94.4%, indicating high reliability of the DCNN-based artifact removal in task and nontask related MEG experiments. The major advantages of the proposed method areas follows: (1) it is a fully automated and user independent workflow of artifact classification in MEG data; (2) once the model is trained there is no need for auxiliary signal recordings; (3) the flexibility in the model design and training allows for various modalities (MEG/EEG) and various sensor types.
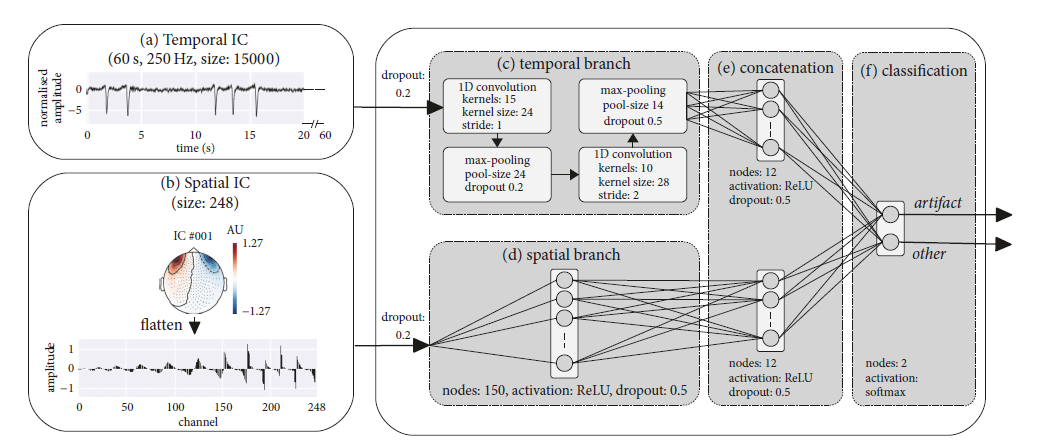
References
Hasasneh, A., Kampel, N., Sripad, P., Shah, N. J., Dammers, J., (2018) "Deep Learning Approach for Automatic Classification of Ocular and Cardiac Artifacts in MEG Data" Journal of Engineering
contact
Dr. Jürgen Dammers
Group Leader: NeuroImaging Data Science
- Institute of Neurosciences and Medicine (INM)
- Medical Imaging Physics (INM-4)
Room 233