AI for Imaging
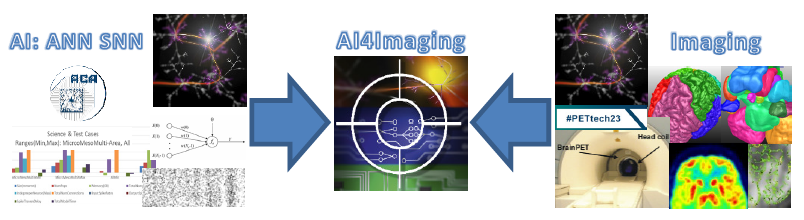
AI4Imaging aims at enhancing the whole data acquisition and data processing chain for energy-efficient, ultra-fast spatio-temporal tomographic brain imaging and analysis at different scales. New concepts and future research will be focussed on artificial & bio-inspired neural networks and their combination with other methods. Algorithms for sensor-near detection (edge computing), image acquisition (correction methods), and image processing (brain parcellation) can be an enabler for new kinds of personalized tomographic investigations in the living brain (e.g., in-vivo connectivity estimations, structure-function relationships on different scales, inter-individual variability & pathologies) with future high-performance medical detector systems (e.g., TOF-PET, fPET, dPEI, MRI, multimodal).
Contact
Dr.-Ing. Gudrun Wagenknecht
Senior Scientist Neuromorphic Computing - Principal Investigator
AI4Imaging for Spatio-Temporal Brain Imaging and Analysis at Different Scales
Energy-efficient, ultra-fast tomographic imaging (e.g., TOF-PET, fPET, dPEI, MRI, multimodal [1-3]) is a perfect application field for AI and NC research since these new computing paradigms can enhance the whole data acquisition and data processing chain [4-9]. Brain imaging at different scales based on artificial & bio-inspired neural networks (e.g., transformers, GNNs, GANs, U-Nets, physics-inspired NNs, bio-inspired SNNs in CIM hardware) can build a very fruitful combination with emerging ultra-fast detector technologies. A first workshop, PETtech23, was organized, where experts from worldwide exchanged the latest advances on detector electronics and AI for data analysis.
New ultra-fast and energy-efficient algorithms for sensor-near detection (edge computing), image acquisition (correction methods), and image processing (brain parcellation) can be an enabler for new kinds of personalized tomographic investigations in the living brain (e.g., personalized in-vivo connectivity estimations, structure-function relationships on different scales, interindividual variability & pathologies) with these future high-performance medical detector systems providing big spatio-temporal (multi-modality) data. An absolut cruicial application would be direct PEI (dPEI), which means 3D PET imaging without image reconstruction. Neuromorphic computing can pave the way to the required 10ps coincidence timing resolution and enable thereby e.g. ultra-fast dynamic fPET measurements and data analysis for personalized in-vivo connectivity estimations.
First experiences with neural network simulation models in neuroscience research were gained within the project “Advanced Computing Architectures” (ACA, 2018-2022). Our objective was to analyze representative models comprising a variety of spatial scales and connectivity structures, neuron and synapse models with and without plasticity dynamics, and to derive requirements for future NC architectures enabling accelerated simulation of ultra-large-scale biological NN models with natural-density, heterogeneous, and plastic connectivity developed in neuroscience research. A comprehensive requirement database for the different network models and their components (structural and functional features and their classification, typical parameterization values and ranges) were developed with regard to relevant properties for future hard- und software designs (e.g., system models, intra- and inter-chip communication concepts).
New concepts and future research will be focussed on artificial & bio-inspired neural networks and combined methods (e.g., graphs, a-priori knowledge,…) for energy-efficient spatio-temporal tomographic image acquisition and processing, and analysis of personalized in-vivo structure-function relationships (e.g., parcellation & functional connectivity, hierarchies, inter-individual variability, plasticity).
This research topic is connected to Neuromorphic-Based Signal Processing, Bio-Inspired Networks & Systems, Interconnecting Spiking Neural Networks, and Neuromorphic Edge Computing Systems.
References
[1] Schaart DR, Physics and technology of time-of-flight PET detectors. Phys. Med. Biol. 66 (2021) 09TR01, 22. doi:10.1088/1361-6560/abee56
[2] Kwon SI, Ota R, Berg E, Hashimoto F, Kyohei Nakajima K et al., Ultrafast timing enables reconstruction-free positron emission imaging, Nature Photonics 15, 914-918 (2021), doi: 10.1038/s41566-021-00871-2
[3] Lecoq P, Morel C, Prior JO, Visvikis D, Gundacker S, et al., Roadmap toward the 10 ps time-of-flight PET challenge, PMB 65 (2020) 21RM01, doi:10.1088/1361-6560/ab9500.
[4] Gong K, Berg E, Cherry SR, and Qi J. Machine Learning in PET: From Photon Detection to Quantitative Image Reconstruction. Proc of the IEEE 108(1) (2020), doi:10.1109/JPROC.2019.2936809.
[5] Debus J, Debus C, Dissertori G, and Götz M, PETNet– Coincident Particle Event Detection using
Spiking Neural Networks, 2024 Neuro Inspired Computational Elements Conference (NICE), doi: 10.1109/NICE61972.2024.10549584
[6] Di Giacomo S, Ronchi M, Borghi G, Schaart DR, et al, Implementing an Integrated Neural Network for Real-Time Position Reconstruction in Emission Tomography With Monolithic Scintillators. IEEE TRPMS 8(5), 501-510 (2024), doi: 10.1109/TRPMS.2024.3378421
[7] Sabri O, Meyer PM, Gräf S, Hesse S, Wilke S, Becker GA, Rullmann M, Patt M, Luthardt J, Wagenknecht G, Hoepping A, Smits R, Franke A, Sattler B, Tiepolt S, Fischer S, Deuther-Conrad W, Hegerl U, Barthel H, Schönknecht P, Brust P. Cognitive correlates of alpha 4 beta 2 nicotinic acetylcholine receptors in mild Alzheimer's dementia. Brain 2018; 141(6): 1840-1854. DOI: 10.1093/brain/awy099
[8] Wagenknecht G, Kaiser HJ, Mottaghy FM, Herzog H. MRI for attenuation correction in PET: methods and challenges. Magn Reson Mater Phy Methods 2013; 26(1): 99-113. DOI: 10.1007/s10334-012-0353-4
[9] Wagenknecht G, Rota Kops E, Mantlik F, Fried E, Pilz T, Hautzel H, Tellmann L, Pichler B, Herzog H. Attenuation Correction in MR-BrainPET with Segmented T1-weighted MR Images of the Patient’s Head – A Comparative Study with CT. IEEE NSS/MIC 2011; Conf. Record: 2261-2266. DOI: 10.1109/NSSMIC.2011.6153858
Copyright ACA-Logo and Icons: Forschungszentrum Jülich GmbH / SBC Lehmann