Advancing High-Resolution Weather Forecasting: Insights from the RAINA Project Meeting
In the first week of March, researchers from Forschungszentrum Jülich (FZJ), Deutscher Wetterdienst (DWD), the European Centre for Medium-Range Weather Forecasts (ECMWF), and the University of Bonn gathered at DWD’s headquarter in Offenbach, Germany, for their first progess project meeting on RAINA (https://raina-project.de/), a collaborative effort led by Michael Langguth (FZJ). RAINA is an initiative focused on improving short-term, high-resolution forecasts of extreme wind and precipitation events with a machine learning-based foundation model for the Earth system. The project works closely with the WeatherGenerator project (https://weathergenerator.eu/), a complementary EU Horizon project contributing digital twin technologies to Destination Earth. By leveraging advanced deep learning methodologies, RAINA seeks to enhance forecasting capabilities critical for disaster prevention and climate change resilience.

Meeting Highlights
A major focus of the discussions was placed on the WeatherGenerator model, whose prototype has recently been made available on github. Christian Lessig (ECMWF) provided an in-depth overview of the model’s structure and architecture. Designed as a community model, it can already ingest different input datasets, such as the ERA5 reanalysis data or satellite observations from SEVIRI, a multispectral camera observing Earth in visible and near-infrared wavelengths, and is capable of generating skilful weather forecasts. The discussion centred on the infrastructure required for sharing training data and how RAINA can integrate into this framework.
One of the key technical topics revolved around input datasets for training. The team deliberated on which datasets would best support RAINA’s objective of improving high-resolution forecasts of extreme wind and precipitation events. Among others, the state-of-the-art ICON-DREAM reanalysis and the RADKLIM dataset were identified as strong candidates. ICON-DREAM provides a multi-year depiction of the global atmospheric state, featuring a refined nest over Europe, while RADKLIM offers over two decades of rain gauge-adjusted precipitation observations at kilometer scale from the German radar network.
In a first prototype application, the project is developing a fully machine learning-based system to generate high-resolution weather forecasts. This approach fuses short-range forecasting with GraphCast, originally developed by Google DeepMind, with a subsequent statistical downscaling step to predict precipitation and wind gusts over Germany at approximately 2 km resolution. During downscaling, not only is the spatial resolution increased, but biases in the GraphCast predictions (Fig. 1)—resulting from the ERA5 dataset on which GraphCast was trained—are also corrected. The development is supported by DWD’s verification suite which enables evaluation using point observations and spatial verification methods through various deterministic and probabilistic scores. A particular focus within RAINA is refining spatial object verification to better account for precipitation-based identification, a necessary step for improving model validation efforts.
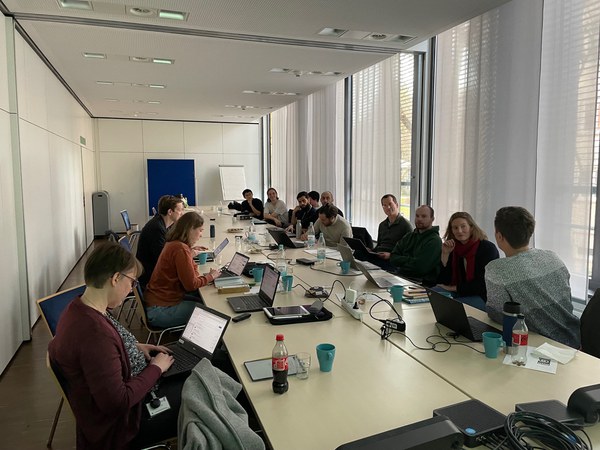
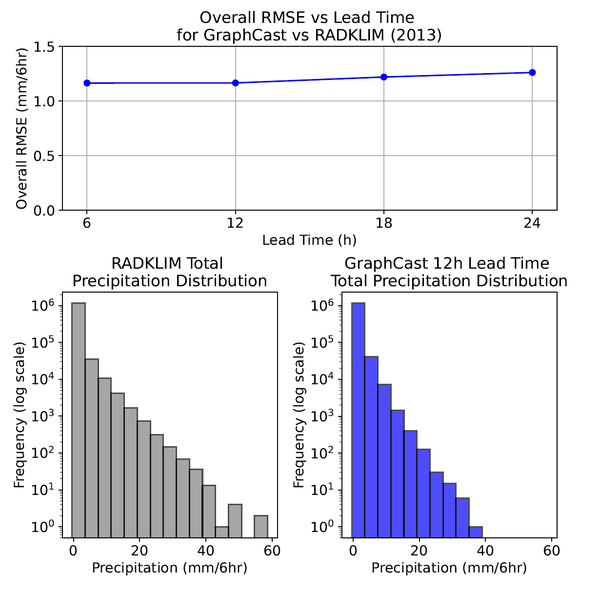
Networking with KI-HopE-DE
Following the RAINA discussions, the team engaged with the sister project KI-HopE-De (AI-based flood forecasting for small catchments in Germany; https://ki-hope.de), which shares a common research call. KI-HopE-DE aims to improve flood forecasting in small and medium-sized river basins, which are particularly vulnerable to extreme rainfall events due to their rapid response times. Traditional hydrological models often struggle to accurately represent their dynamics, leading to significant uncertainties in flood predictions. By leveraging AI, KI-HopE-DE seeks to develop a robust and flexible forecasting system for catchments smaller than 500 km². The project will compile a comprehensive hydro-meteorological dataset for training and validating AI-based models, with a primary focus on short-term forecasts (less than 48 hours).
The discussion covered the strengths and weaknesses of different datasets and modelling approaches. Notably, KI-HopE-DE researchers highlighted the use of Long Short-Term Memory (LSTM) models, a type of recurrent neural network well-suited for time-series forecasting, as they can effectively capture long-range dependencies in hydrological processes. Both projects share an interest in probabilistic modelling, providing a measure of uncertainty that is crucial for risk assessment.
A particularly promising avenue for collaboration emerged in evaluating how improved precipitation forecasts from RAINA could promote better flood predictions. This cross-project exchange reinforced the importance of interdisciplinary cooperation in advancing weather prediction science.
The meeting concluded on a positive note, with fruitful discussions paving the way for future advancements in high-resolution weather forecasting.
Contact
Prof. Dr. Martin Schultz
Head of research group Earth System Data Exploration and co-lead of division Large Scale Data Science, University professor in Computational Earth System Science at the University of Cologne PI in Helmholtz Information Program 1, Topic 1 (Topic Board Member)
- Institute for Advanced Simulation (IAS)
- Jülich Supercomputing Centre (JSC)
Room 4010