TerraMind: A Breakthrough Multimodal Model for Global-Scale Earth Observation
The FAST-EO project has released the TerraMind AI foundation model, the first any-to-any generative, multimodal foundation model for Earth observation, integrating satellite imagery, elevation data, land use maps, and natural language descriptions. TerraMind was pretrained both on token-level and pixel-level data across nine modalities using over 500 billion tokens on the JUWELS Booster HPC system at JSC.
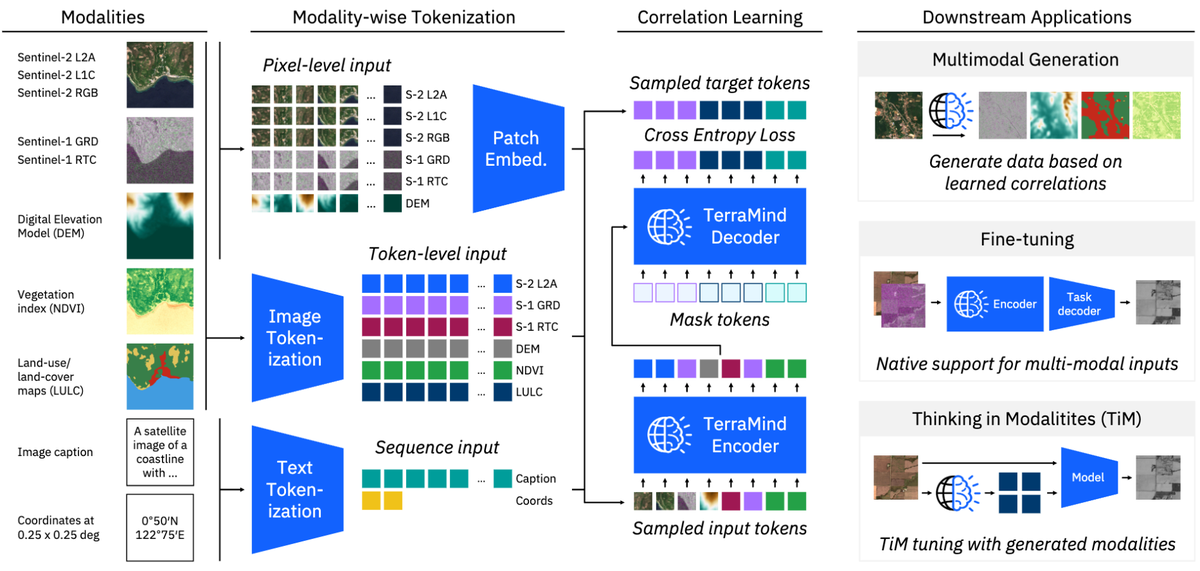
TerraMind achieved top performance on the PANGAEA benchmark, outperforming all previously published geospatial foundation models across diverse Earth observation tasks. Model weights are now available on Hugging Face for fine-tuning and the paper is available on arXiv.
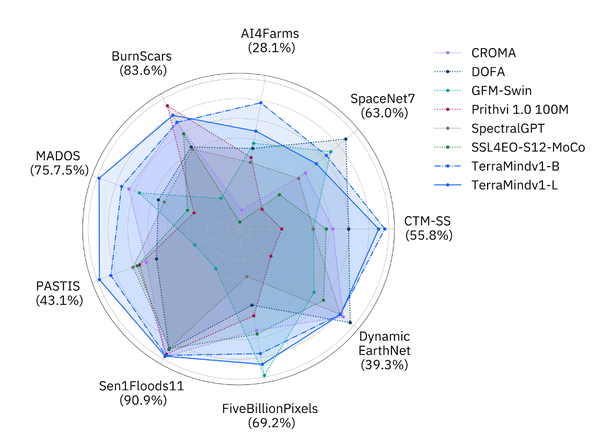
JSC’s SDL (Simulation and Data Lab) AI and ML for Remote Sensing aims to increase the adoption of interdisciplinary research combining remote sensing applications, large-scale AI, and high-performance and innovative computing. Being an integral part of the work on TerraMind is an important step towards that aim.
Dr. Rocco Sedona said, “With an eye on the future, by leveraging the JUPITER AI Factory—a cornerstone of Europe’s AI infrastructure—we are committed to providing the Earth observation community with powerful, transformative tools that will drive their downstream applications forward.” Prof. Gabriele Cavallaro added “It’s remarkable to witness how rapidly AI research and achievements are evolving across numerous domains. Geospatial foundation models are emerging as the backbone of geoscience and remote sensing, driving progress in addressing the many challenges we face, particularly those tied to climate change.”
You can find the full paper here: https://arxiv.org/pdf/2504.11171
For more information about research and work activities of the SDL AI and ML for Remote Sensing, visit https://www.fz-juelich.de/en/ias/jsc/about-us/structure/simulation-and-data-labs/sdl-ai-ml-remote-sensing
Contacts at JSC
Dr. Rocco Sedona
Deputy Head of Simulation and Data Lab (SDL) Artificial Intelligence and Machine Learning for Remote Sensing
- Institute for Advanced Simulation (IAS)
- Jülich Supercomputing Centre (JSC)
Room 401
Prof. Dr. -Ing. Gabriele Cavallaro
Head of Simulation and Data Lab (SDL) Artificial Intelligence and Machine Learning for Remote Sensing
- Institute for Advanced Simulation (IAS)
- Jülich Supercomputing Centre (JSC)
Room 3001