ESDE Group Expands into Three Teams
Since the beginning of the year, the Earth System Data Exploration (ESDE) group has been restructured into three dedicated teams: Data Infrastructure and Workflows (DIW), WeatherAI, and ClimateAI. This change reflects the increasing number of researchers within our group and the growing importance of our work in leveraging deep learning and high-performance computing for weather, climate, and air quality studies. Notably, the former "Deep Learning for Weather and Air Quality" team has now been split into WeatherAI and ClimateAI, both of which still incorporate air quality aspects in their research.
The teams are led by:
- Data Infrastructure and Workflows: Sabine Schröder
- WeatherAI: Michael Langguth
- ClimateAI: Savvas Melidonis
The ESDE group explores cutting-edge deep learning methods and large-scale data workflows to analyse and forecast atmospheric phenomena, with a strong focus on air quality and weather. Our work enhances our ability to predict and mitigate the impacts of extreme weather events, protect ecosystems, and support science-based decision-making. We collaborate with many international partners to drive advancements in machine learning and Earth system science, contributing significantly to major European initiatives such as AtmoRep and WeatherGenerator.
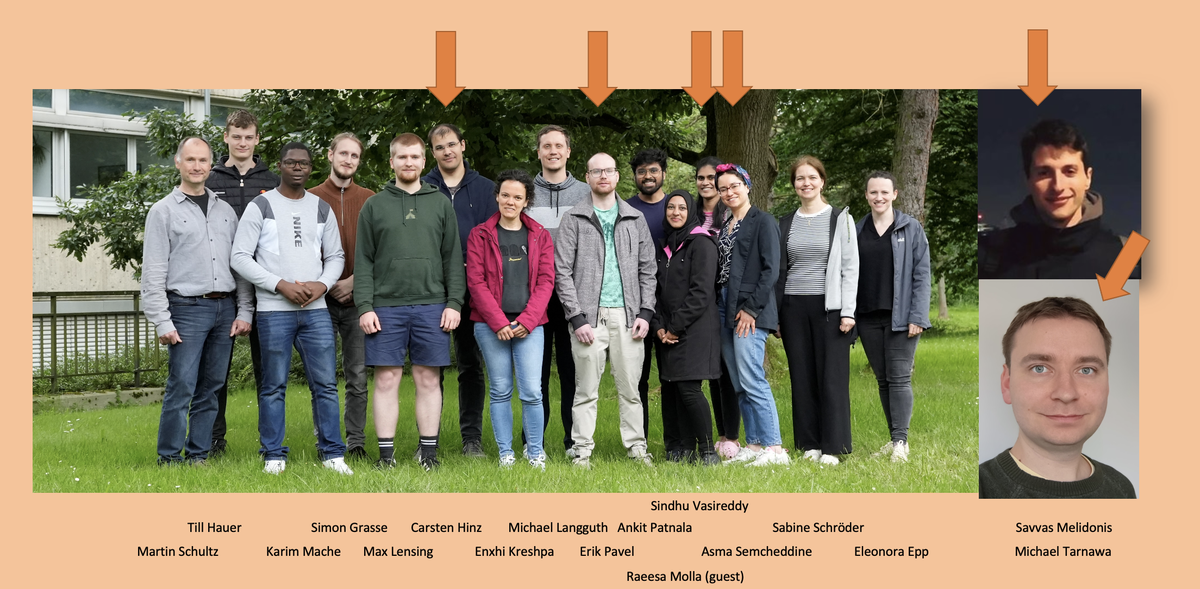
Data Infrastructure and Workflows (DIW)
The DIW team specialises in developing state-of-the-art data infrastructure and analytical tools for air quality and meteorological data. Their work ensures that harmonised, quality-controlled datasets are openly accessible following FAIR principles. The team plays a pivotal role in projects such as:
- TOAR Database Infrastructure (toar-data.fz-juelich.de, toar-data.org): A large-scale database for air quality and meteorological measurements.
- WarmWorld (https://www.warmworld.de): Improving Earth system simulations and facilitating access to climate data through federated systems.
WeatherAI
WeatherAI focuses on deep learning-driven weather forecasting, developing foundation models that enhance predictability, particularly for extreme events. By integrating machine learning with vast environmental datasets, the team is pushing the boundaries of atmospheric modelling. They actively contribute to:
- WeatherGenerator (https://weathergenerator.eu): A four-year EU project building on the AtmoRep initiative to advance machine learning in weather prediction.
- RAINA (https://raina-project.de): Focused on extreme weather events such as heavy precipitation and flooding, contributing to the WeatherGenerator.
ClimateAI
ClimateAI explores the application of deep learning to climate modelling, particularly in developing AI-based foundation models for long-term climate simulations. Their research aims to achieve atmosphere-ocean-sea-ice coupling and enhance seasonal-to-decadal predictions. Key projects include:
- HClimRep (https://hclimrep-project.de): Extending machine learning methodologies to climate-scale modelling within the EU WeatherGenerator framework.
The restructuring of ESDE into these specialised teams strengthens our ability to address pressing scientific challenges in Earth system science. Through close collaboration and interdisciplinary expertise, we continue to shape the future of weather and climate modelling.