SDL Electrons and Neutrons
SimDataLab Mission
The Simulation and Data Laboratory Electrons & Neutrons (SDLen) forms a bridge between experiment and simulation with a special focus on data management and data analytics using high-performance computing and methods from the field of artificial intelligence. SDLen offers high-level technology support to users in electron microscopy, neutron scattering and related fields. Besides that, the lab hosts selected research projects targetting the development of novel methods and the usability of state-of-the-art technology.
Naturally, SDLen also forms a bridge between the Jülich Supercomputing Centre (JSC) and the two mainly experimentally focussed institutes: Ernst Ruska-Center for Electron Microscopy (ER-C) and the Jülich Center for Neutron Science (JCNS). With lab members being employees of the three organizational units (JSC, ER-C and JCNS), the lab acts as an incubator for new approaches in the field of data analysis and data management.
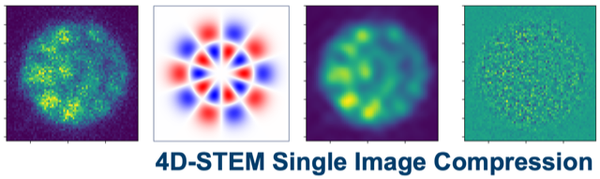
Introduction
The approach of the Simulation and Data Laboratory Electrons & Neutrons (SDLen) is to enable a thorough understanding of materials and nanoscale structures from two sides:
Using customized data analysis methods onto experimental data obtained from sophisticated probing methods (such as TEM, STEM, EDX, XRCD, SAX, SANS) allows to draw conclusions about the nature of the investigated sample.
This is augmented by the simulation approach: Realistic models of the sample are constructed in-silico to verify the experimental findings on a qualitative and quantitative level. This includes the application of electronic structure methods, (ab-initio or classical) molecular dynamics, micromagnetic or multi-slice simulations, to name a few.
Lab members
The SDLen staff constitutes of members of the Ernst Ruska Centre for Microscopy with Electrons (ER-C), the Jülich Centre for Neutron Science (JCNS) and the Jülich Supercomputing Centre (JSC) at Forschungszentrum Jülich.
Within the Program-oriented Funding (PoF IV) of Helmholtz Information, this group contributes to Program 1 “Engineering Digital Futures”, Topic 1 “Enabling Computational- & Data-Intensive Science and Engineering”.
Activities
The activities of SDLen include but are not limited to:
- The development of LiberTEM, a python-based data analysis package for electron microscopy data
- Supporting researchers in the optimization and modernization of simulation and analysis codes to run efficiently on state-of-the-art supercomputing architectures;
- Driving research for high-key analysis methods using artificial intelligence, such as holography;
- Development of simulation packages for the all-electron description of nanoscale structures;
- The maintenance of a platform for electronic lab books and other data management tools;
- Supporting researchers to get HPC benchmarks and write compute time applications;
- The maintenance of a knowledge base for the usage of HPC resources;
- The organization of training events for Machine Learning Methods;
Collaborations
Heinz Maier-Leibnitz Zentrum für Neutronenstreuung Garching https://mlz-garching.de/
SaxonyAI https://saxony.ai/
Peter-Grünberg Institute (PGI-1) https://www.fz-juelich.de/de/pgi/pgi-1
Joint Lab Virtual Materials Design https://www.fz-juelich.de/de/pgi/pgi-1/forschung/joint-lab-vmd
Projects
VIPR (BMBF ErUM-Data)
EOSC Data Commons
IMPRESS
AIDAS
CIO Budget
Helmholtz Innovation Pool MDMC
DAPHNE
Helmholtz Innovation Pool ELLIPSE