Scientific Machine Learning in Quantum Materials
In the last decade, the proliferation of materials simulations has opened the way to a massive flow of data testified by the publication of tens of thousands of articles per year only in the field of Density Functional Theory.

This proliferation created the need to create a systemic approach to store not only the results of a simulation but the full step-wise workflow that end up in a specific result. This need has been encoded in international efforts like the Nomad Lab or the Materials Cloud database.
By now this field expanded beyond the handling of large amount of data to include methods for the exploitation of them rooted in Machine and Deep Learning techniques. Overall, the community effort in this direction created the relatively new field of Materials Informatics. Despite the enormous advances towards a full digitalization of the simulation process from the single atoms to the properties of the Digital Twin of a Material, the mere size of the quantum chemistry surrogate space leaves many challenges still unanswered.
SDL Quantum Materials is active in the field of Scientific Machine Learning with the objective to develop models of data analysis where the final result has a truly explainable nature. For instance in one of our most recent work, we have shown that, through a combination of standard techniques (KRR + LASSO + sparsification), we can add analytical terms expressing the excess of Enthalpy of formation for solid solutions of Lanthanides phosphates.
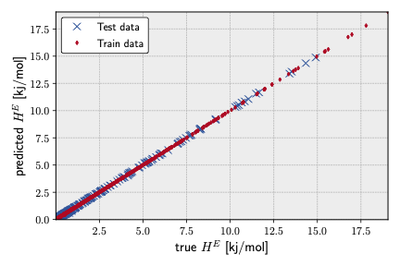
Regression for the degree 3 polynomial Kernel (right). The scatter plots corresponds to a trained model whose hyperparameters have been already fitted. Degree 3 polynomial the best fit: λ = 104.74, γ = 105.79, c = 100.37 with an MAE of 0.0090 kJ/mol.
In addition, the SDL Quantum Materials provides support for the data management and data usage on the JSC supercomputing clusters. In practice this implies a sustained support for existing platforms such as AiiDA, which is already been integrated within the Jupyter environment at the JSC. This effort is part of the Helmholtz Joint Lab Virtual Material Design of which SDLQM is an active member.