Open Access Publication of the Month – Chadi Barakat (JSC) et al.
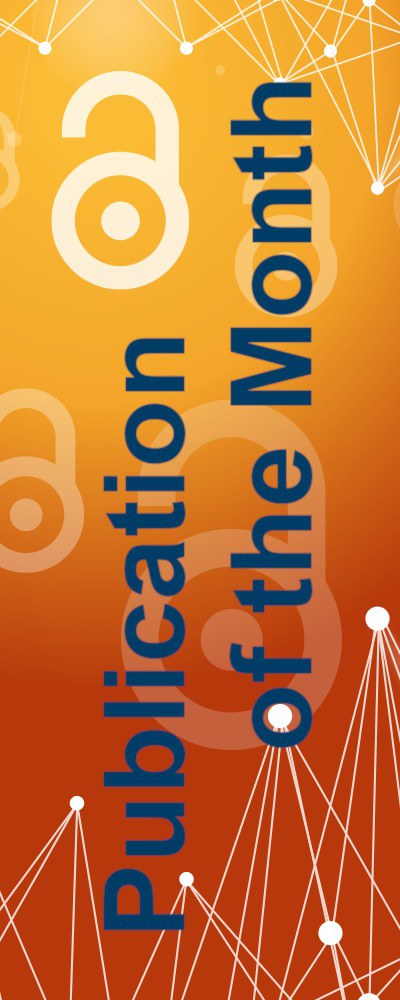
8 February 2023
To encourage us all to broaden our horizons, each month the Central Library selects one open access publication from the JuSER publications portal to be featured in the newsletter of Forschungszentrum Jülich.
In this month’s open access publication, Chadi Barakat (JSC, University of Iceland (UI)), Marcel Aach (JSC, UI), Sigurður Brynjólfsson (UI), Andreas Schuppert (University Hospital RWTH Aachen), Sebastian Fritsch (JSC, University Hospital RWTH Aachen), and Morris Riedel (JSC, UI) write about the development and application of a modular machine learning and data science platform on which diagnostic ML models can be developed, tested, and deployed.
The publication is the culmination of work on retraining the COVID-Net model developed by Wang et al. for rapid COVID-19 diagnosis using newly obtained data from industry partner E*Healthline in the EuroCC project’s EOSC fast-track grant for COVID-19 research.
The initial – previously published – results showed that COVID-Net needed to be retrained with the new data before its prediction performance was within an acceptable range. It was also found that performance was only marginally acceptable, even after several rounds of trial and error, to select a combination of parameters with which to train the model. In order to streamline this process, the authors set up a platform on which they could work on completing this specific task, but which could easily be adapted to later machine learning tasks. They performed parallelized hyperparameter tuning on the platform to uncover the combination of parameters through which the COVID-Net model had the best performance on the data. They then used those parameters to retrain the model at full scale, which allowed them to highlight both the improvement in performance and, more importantly, the speed-up that can be achieved by scaling up the available compute resources. Since the platform uses open access data and open source software, it could easily be ported to commercial cloud computing platforms. The authors believe that this opens up the opportunity for medical institutions to develop their own diagnostic models, which can then simply be exported as standalone modules and easily implemented where necessary, especially under time-sensitive circumstances such as during the early stages of the COVID-19 pandemic.
The open access publication entitled "Analysis of Chest X-ray for COVID-19 Diagnosis as a Use Case for an HPC-Enabled Data Analysis and Machine Learning Platform for Medical Diagnosis Support" was published in the journal Diagnostics in a special issue on “Artificial Intelligence in Image-Based Screening, Diagnostics, and Clinical Care of Cardiopulmonary Diseases”.
JuSER publications portal – Analysis of Chest X-ray for COVID-19 Diagnosis as a Use Case for an HPC-Enabled Data Analysis and Machine Learning Platform for Medical Diagnosis Support
Internet – Institute for Advanced Simulation (IAS) – Jülich Supercomputing Centre (JSC)