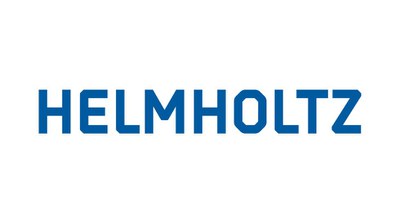
Laufzeit
October 2020 bis September 2023
Kontakt
Prof. Dr. Uwe Rascher
Gebäude 06.2 / Raum 406b
+49 2461/61-2638
E-MailFluoMap
Machine Learning-based Fluorescence Retrieval from Optical Satellite Data
Fluorescence is an important biophysical parameter linked to gross primary productivity of plants and allows the detection of vegetation stress in very early stages. Offering great potential in this field, modern airborne and spaceborne imaging spectrometers are now sensitive enough to record the effect of fluorescence superimposed with signal responses from the earth surface and the atmosphere. For the first time, we propose the development of a Machine Learning (ML) approach to derive solar-induced fluorescence maps from imaging spectrometer measurements over extended areas. This is a Helmholtz opportunity, as for this purpose combined hyperspectral data from an airborne sensor (HyPlant) and from space (DESIS sensor on ISS) is available only to the project partners.
The proposed end-to-end system is designed to derive fluorescence maps directly from the pure measurements taking into account the complex atmospheric influences on the signal as well as sensor specific uncertainties. To solve this ill-posed inversion problem (Deep) Neural Networks are trained with not only with measured data, but also simulated data calculated by physical forward models. A series of deliverables will be released that can be used by the Earth Observation scientific community.
Contacts IBG-2