Artificial Intelligence in Brain Research
Prof. Timo Dickscheid, head of the Big Data Analytics working group, knows the opportunities and challenges associated with AI.
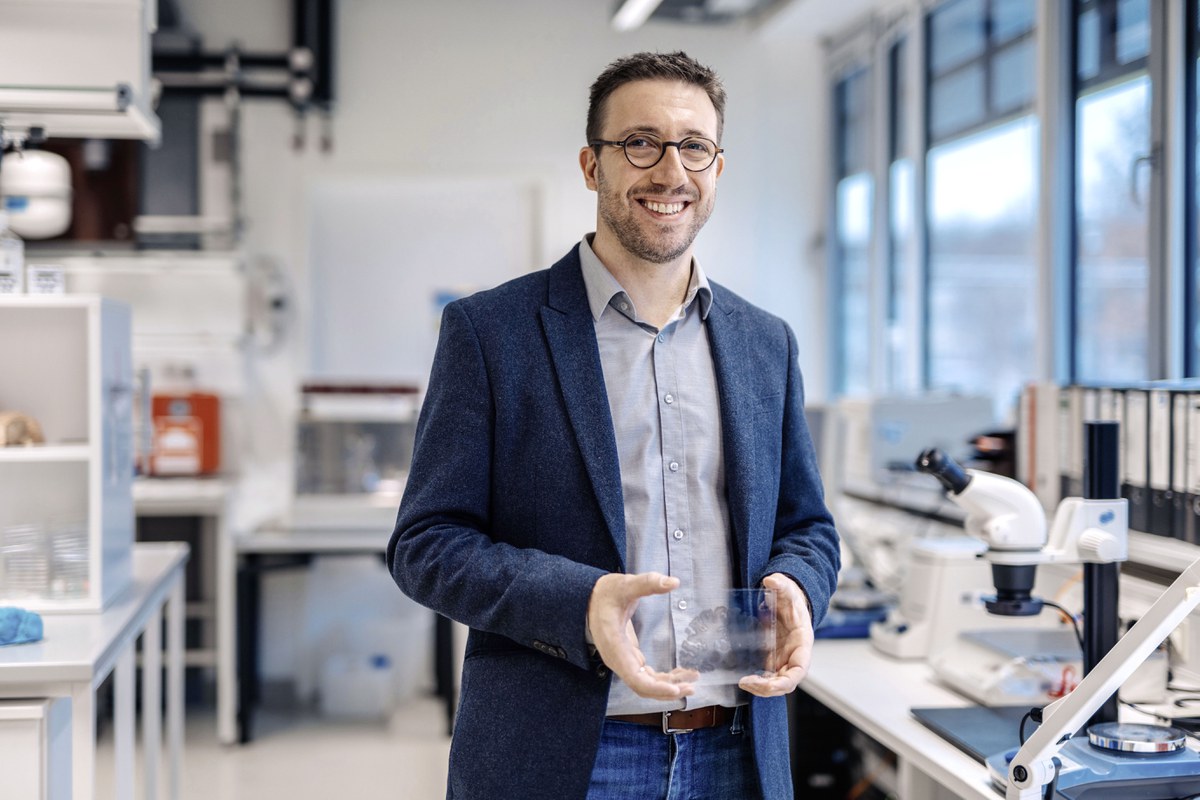
There are many areas of application for artificial intelligence (AI) in brain research. It can improve the work of researchers, but also makes new things possible, for example in brain image analysis. At INM-1, we are currently developing an AI method to deduce where cell bodies are located directly from nerve fibre images. This is something we have not previously been able to do. AI is already helping us to reconstruct countless individual brain slices on the computer much more accurately and reliably in three dimensions, and to also digitally “fill in” any missing or damaged brain slices.
There are some things that AI can’t necessarily do better, but that it can do much faster. For example, it usually takes a neuroanatomist several months to comprehensively map an area of the brain. We were able to show for some brain areas that AI can do good mapping work in minutes. This is not about us wanting to replace neuroanatomists, but it does mean we can create brain atlases much faster. Together with Canadian partners in the HIBALL project, we are also using findings from ‘real’ networks in the brain for artificial neural networks in our AI – and vice versa.
Admittedly, AI also brings huge challenges. INM generates several terabytes of research data every day. It has so far been difficult to keep up with this pace in our AI analyses. As we are constantly improving our methods, we sometimes have to reanalyse previous data. The workflow from the microscope to the online atlas is not yet fully automated, but we are working on it. We also need to ensure that AI results are correct in the first place. We can check many things by having researchers carry out the same steps manually in random samples for comparison. We are also planning separate AI programmes that detect “surprises” in the data stream and then raise the issue.
At the same time, we are researching how AI models can be optimally trained with as many images as possible, even if they were not previously prepared for training by humans. The wealth of data we have amassed at INM over decades is worth its weight in gold. We also want to find out whether and how brain images available on the Internet, which may be of poorer quality, can help us as additional ‘training fodder’. This can’t be done with pen and paper; we have to try it out with huge amounts of data. The new exascale supercomputer JUPITER will help us a lot in this regard.
Originally published in the employee magazine "intern" of Forschungszentrum Jülich.
Editor: Hanno Schiffer
Contact
Prof. Dr. Timo Dickscheid
Working Group Leader "Big Data Analytics"
- Institute of Neurosciences and Medicine (INM)
- Structural and Functional Organisation of the Brain (INM-1)
Room 4009
Media Contact
Erhard Zeiss
Wissenschaftlicher Kommunikationsreferent
- Institute of Neurosciences and Medicine (INM)
- Structural and Functional Organisation of the Brain (INM-1)
Room 3033